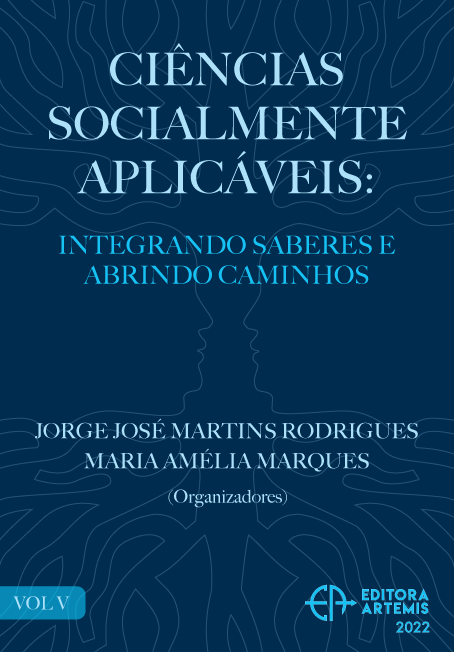
STATISTICAL ANALYSIS OF CONVERGENCE FOR NON-LINEAR OPTIMIZATION ALGORITHMS IN CALL CENTERS PROBLEMS
A otimização de call center não é um problema fácil de resolver, devido à complexidade dos modelos matemáticos que derivam das fórmulas Erlang. Essa complexidade é transferida para modelos de otimização, que na maioria dos casos, estão em conformidade com funções objetivas não lineares, não convexas e não derivadas. Como em todas as áreas de pesquisa de operações, resolver esses problemas exige algoritmos eficientes, rápidos e precisos. Na maioria dos problemas de otimização não linear com restrições, o pesquisador enfrenta a dificuldade de verificar e validar o funcionamento correto do algoritmo. Em muitos casos, a complexidade computacional dificulta a estimativa do resultado da força bruta. A maneira usual é ter um conjunto de problemas semelhantes ou equivalentes bem definidos e com métricas de resultados conhecidos com as quais podem ser contrastadas. O problema é que você nem sempre tem esses repositórios. A necessidade de ter uma estratégia para validar a convergência e a precisão de uma sequência numérica gerada por um algoritmo de otimização se torna imperativo. Este trabalho descreve um método estatístico para avaliar rapidamente a convergência e a precisão dos algoritmos que geram sequências numéricas na aproximação de um resultado.
STATISTICAL ANALYSIS OF CONVERGENCE FOR NON-LINEAR OPTIMIZATION ALGORITHMS IN CALL CENTERS PROBLEMS
-
DOI: 10.37572/EdArt_25082263715
-
Palavras-chave: Otimização não linear, convergência de algoritmos de otimização, otimização não linear inteira, otimização de call center
-
Keywords: Non-linear optimization, Convergence of optimization algorithms, Integer Nonlinear Optimization, Call Center Optimization
-
Abstract:
Call center optimization is not an easy problem to solve, due to the complexity of the mathematical models that derive from Erlang formulas. This complexity is transferred to optimization models, which in most cases, conform to non-linear, non-convex and non-derivable objective functions. As in all areas of Operations Research, solving these problems demands efficient, fast and accurate algorithms. In most nonlinear optimization problems with constraints, the researcher is faced with the difficulty of verifying and validating the correct functioning of the algorithm. In many cases, computational complexity makes it difficult to estimate the result by brute force. The usual way is to have a set of similar problems or well-defined equivalents, and with known results metrics with which it can be contrasted. The problem is that you don't always have these repositories. The need to have a strategy to validate the convergence and precision of a numerical sequence generated by an optimization algorithm becomes imperative. This work describes a statistical method to quickly evaluate the convergence and accuracy of algorithms that generate numerical sequences in the approximation of a result.
-
Número de páginas: 16
- Ángel Rubén Barberis
- Lorena Elizabeth Del Moral Sachetti