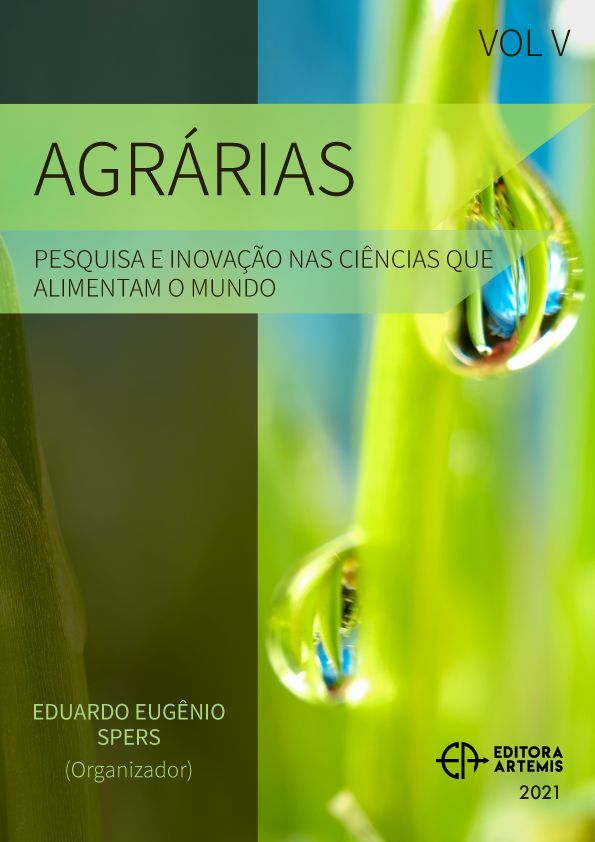
PREDICCIÓN DE LA IRRADIACIÓN SOLAR GLOBAL DIARIA MEDIANTE REDES NEURONALES ARTIFICIALES EN LA PENÍNSULA IBÉRICA
Este artículo, se centra en la predicción de la irradiación solar global diaria horizontal, por ser el caso más interesante en la meteorología agrícola, por ejemplo, en las previsiones de necesidades de riego, utilizando la técnica de las redes neuronales artificiales (RNAs) de la inteligencia computacional, a partir de variables accesibles en las estaciones agrometeorológicas. El lugar donde fueron medidos los datos, utilizados para entrenar las RNAs, caracterizan donde se pueden volver a utilizar este tipo de modelos, en este estudio fueron las estaciones meteorológicas de la red SIAR en Castilla y León (España), en concreto la situada en Mansilla Mayor (León), durante los años 2004-2010. Los modelos RNAs se construyeron en la entrada con los datos medidos de irradiación solar global diaria de uno, dos y tres días anteriores, añadiendo el día del año J(t)=1..365, para predecir su valor el día siguiente. Los resultados obtenidos, validados durante el año 2011 completo RMSE=3,8012 MJ/(m2d), concluyen que las RNAs estudiadas mejoran los métodos clásicos comparados: 1) año típico CENSOLAR RMSE=5,1829 MJ/(m2d), 2) media móvil ponderada con la autocorrelación parcial de 11 días de retardo RMSE=3,9810 MJ/(m2d), 3) regresión lineal sobre el valor del día anterior RMSE=4,2434 MJ/(m2d), 4) año típico Fourier utilizado el 1er armónico RMSE=4,2747 MJ/(m2d), y 5) las matrices de transición de Markov para 33 estados posibles RMSE=4,3653 MJ/(m2d). Durante los días de cambio brusco en el nivel de irradiación solar, se observan los mayores errores de predicción. Se plantea utilizar en la entrada otras variables para mejorar la eficacia del modelo RNA. Una de las variables probadas fue el índice de claridad diario Kt=H/H0, resultando una mejora RMSE=3,7703 MJ/(m2d).
PREDICCIÓN DE LA IRRADIACIÓN SOLAR GLOBAL DIARIA MEDIANTE REDES NEURONALES ARTIFICIALES EN LA PENÍNSULA IBÉRICA
-
DOI: 10.37572/EdArt_2904213478
-
Palavras-chave: predicción, insolación, evapotranspiración, agrometeorología, inteligencia computacional.
-
Keywords: prediction, insolation, evapotranspiration, agrometeorology, computational intelligence.
-
Abstract:
This article focuses on the prediction of the horizontal daily global solar irradiation, as it is the most interesting case in agricultural meteorology (e.g., in forecasting irrigation needs), using the technique of artificial neural networks (ANNs) of computational intelligence, based on accessible variables at agrometeorological stations. The place where the data were measured, used to train the ANNs, characterize where this type of model can be used again, in this study it was the meteorological stations of the SIAR network in Castile and León (Spain), specifically the one located in Mansilla Mayor (León), during the years 2004-2010. ANNs models were built at the entrance with the measured data of daily global solar irradiation of one, two and three previous days, adding the day of the year J(t)=1..365, to predict its value the following day. The results obtained, validated during the full year 2011 RMSE=3.8012 MJ/(m2d), conclude that the ANNs studied improve the classic compared methods: 1) typical year CENSOLAR RMSE=5.1829 MJ/(m2d), 2) weighted moving average with the partial autocorrelation of 11 days of delay RMSE=3.9810 MJ/(m2d), 3) linear regression on the value of the previous day RMSE=4.2434 MJ/(m2d), 4) typical Fourier year used the 1st harmonic RMSE=4.2747 MJ/(m2d), and 5) the Markov transition matrices for 33 possible states RMSE=4.3653 MJ/(m2d). During the days of sudden change in the level of solar irradiation, the largest prediction errors are observed. It is proposed to use other variables in the input to improve the efficiency of the ANN model. One of the variables tested was the daily clearness index Kt=H/H0, resulting in an improvement in RMSE=3.7703 MJ/(m2d).
-
Número de páginas: 34
- Francisco Javier Diez
- Luis Manuel Navas Gracia
- Andrés Martínez Rodríguez
- Adriana Corrêa Guimarães
- Leticia Chico Santamarta